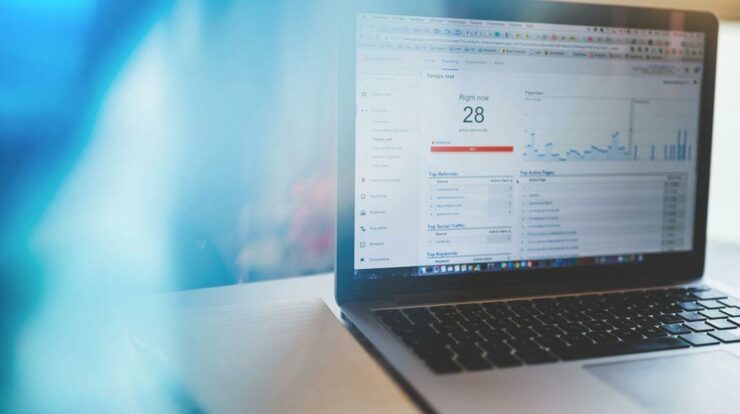
The number of organizations that leverage Data Science to extract knowledge and insights from the data continues to increase. While data science refers to using data analytics tools, there is also a human element. Data scientists are responsible for making sense of data and providing actionable insights.
The human element makes the entire process prone to specific errors due to data science bias. Today we will take a closer look at bias in data science, explore some examples, and help you discover ways to prevent bias and always draw correct conclusions.
What is data science bias?
To understand data science bias, you first need to understand what exactly bias means. According to Merriam-Webster, bias is defined as:
“Systematic error introduced into sampling or testing by selecting or encouraging one outcome or answer over others.”
In data science, it refers to a deviation from the expectation in the dataset. It almost always results in an error that can significantly impact your decision-making process. For instance, you can base your business decision on inaccurate insights because the analysts or data scientists didn’t see the whole picture or consider other data points.
Data science bias can take many shapes and forms. Here are the five types of biases that regularly occur in data science and how you can avoid them.
Availability bias
Availability bias is one of the most common data science biases analysts have. It’s rooted in data scientists’ belief that the data they can easily access is the most relevant data. Drawing insights from readily available data can have dire effects and can prevent analysts from taking into account other essential data points.
The easiest way to prevent availability bias is to stay tuned to the latest digital marketplace trends. Enriching the readily available data with structured, up-to-date, relevant, and accurate data sets will enable analysts to access more data points.
Plus, you can increase standards for running analytics. For instance, you can specify the minimum number of data points and the sample size to be used.
Selection bias
Next, we have the selection bias. The selection bias occurs when analysts use an incomplete data sample. In other words, the data set doesn’t completely represent the object of the analysis. For instance, you have access to firmographic data, but it doesn’t come with information about companies’ revenue and trends.
When it comes to preventing selection bias, there are a couple of strategies you can use. You should structure and standardize the collection of data to ensure that you collect all the vital information for every entity.
You should also ensure that no incomplete entity ends up in your data set. Finally, regularly updating your data while using the above-mentioned strategies can help you prevent selection bias.
Confirmation bias
Confirmation bias has a lot to do with human nature. Data scientists and analysts are humans, and as humans, they are susceptible to letting past experiences and expectations cloud their perception and judgment. Additionally, their personal opinions and views can distort insights.
Confirmation bias doesn’t have anything to do with your data. In fact, it can distort even the most complete and accurate datasets because analysts draw insights from the data that aligns with their expectations or theory. Even if a data point doesn’t fit the analysts’ preconceived notion, they won’t take it into account.
The best way to mitigate this risk is to ensure at least two analysts are working on your data. You can also organize training to help your analysts understand confirmation bias.
Recall bias
Drawing the most accurate insights has a lot to do with the analysts’ ability to consider the patterns and trends they’ve identified in the past. Recall bias refers to analysts’ inability to leverage past insights when drawing the insights from the latest report.
Historical information is vital as it can help analysts better understand current developments. The most efficient way to avoid recall bias is to analyze the current data sets with the previous insights in mind.
Survivorship bias
Survivorship bias is probably the most dangerous one in terms of providing insights with catastrophic consequences. It refers to focusing only on the successful entities in the data set while completely ignoring the failures.
For instance, your analysts can look at firmographics and only focus on thriving, relevant companies while paying no attention to companies that failed, or focusing on repeat customers with no interest in customers that stop purchasing products or services from a company.
The only way to mitigate the risk of survivorship bias is to ensure that your analysts look at all data entities, including examples of success, mediocre performance, and failures.
Conclusion
When it comes to the factors able to hinder the accuracy of the data analytics results, data science bias is probably the one with the most impact. Knowing all the potential biases, your data analysts can make can help you develop strategies to prevent them and ensure you make your business decisions based on accurate and up-to-date insights.